I. Introduction
Data is more than just numbers. While quantitative methods such as surveys can measure customer satisfaction, emotional state, and other internal phenomena, these instruments don’t tell us the reasoning behind those answers or how a business can better support its customer needs.
Qualitative research is done to understand the ‘why’ behind decisions. However, how do we know if our qualitative findings are trustworthy and applicable?
While you can measure numerical data through validation, editing, and coding, this standardized approach doesn't apply to qualitative data's descriptive and conceptual nature. Verifying the accuracy of your findings differs significantly from quantitative methods.
Fortunately, there are many ways to ensure data quality in your results. This in-depth guide will discuss the various aspects of data quality and give you a better understanding of how you can collect and organize your qualitative data for improved decision-making.
II. Defining Data Quality
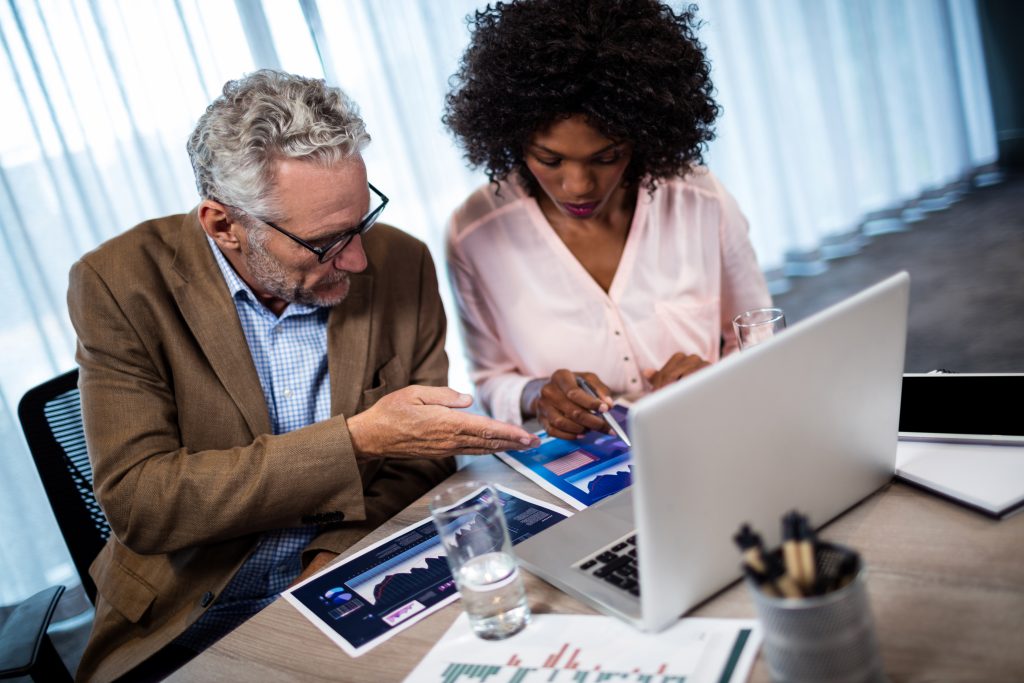
Data quality indicates how accurate a given set of research findings are. This attribute directly impacts a company's ability to plan and make decisions based on its study sample.
Accurate market research requires you to gather quality data from research participants. This data can be deemed ‘high-quality’ if it possesses these four attributes:
1) It satisfies its intended purpose: The data must fulfill its intended purpose by providing insights relevant to the research goals. If the data is unable to address the research questions or objectives, it lacks value.
2) It is closely related to the construct of the project: The research findings should directly correlate with the variables or topics being studied.
3) It has built-in controls to ensure it is free from bias: The data collection process includes parameters to reduce biases that could distort your findings.
4) It verifies that research respondents have been vetted for qualification: The participants are genuinely representative of your project’s target population and can provide meaningful feedback.
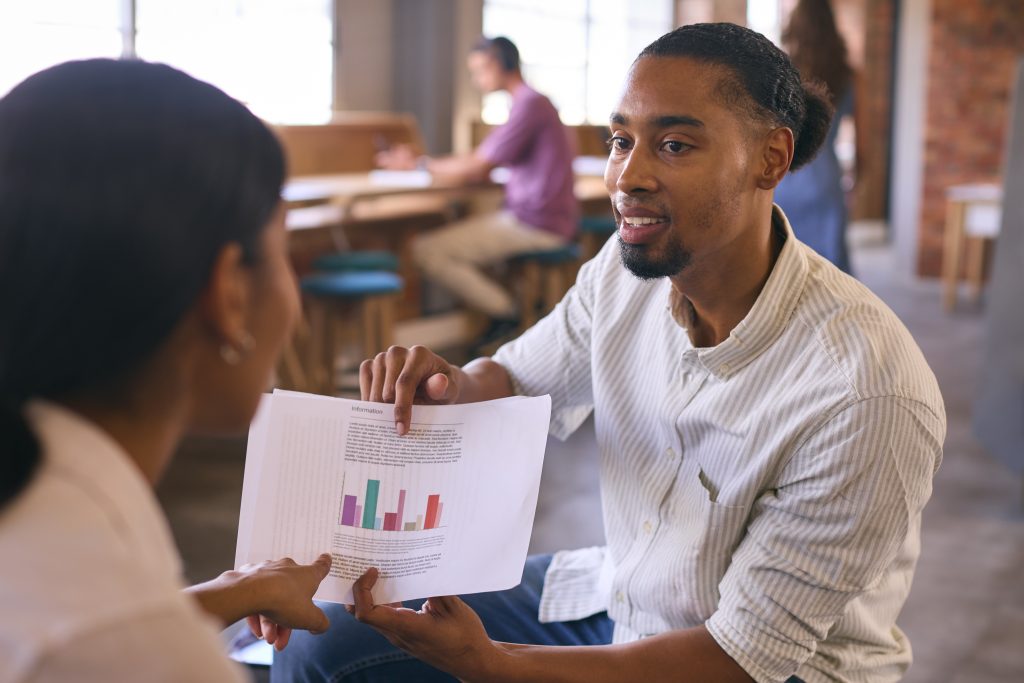
For instance, a researcher at a food and beverage company is tasked with collecting data from a consumer product testing experience to guide future product development. In this case, the data is considered high-quality because:
- It helped the company understand what consumers want from the product, providing actionable insights for future product innovations (satisfies intended purpose).
- It was directly tied to specific consumer preferences regarding taste, packaging, and overall product satisfaction (relevant to construct).
- The data collection process included randomized participant selection and consistent testing conditions, ensuring that responses are authentic and reliable (built-In controls to minimize bias).
- Participants were carefully screened and vetted to fit the project’s respondent criteria (vetted for qualification).
Data quality influences the quality of the insights gained from the research and, therefore, the effectiveness of the decisions made. Without meeting these criteria, your data could lead to flawed conclusions, undermining the value of the research as a whole.
III. Why is Data Quality Important?
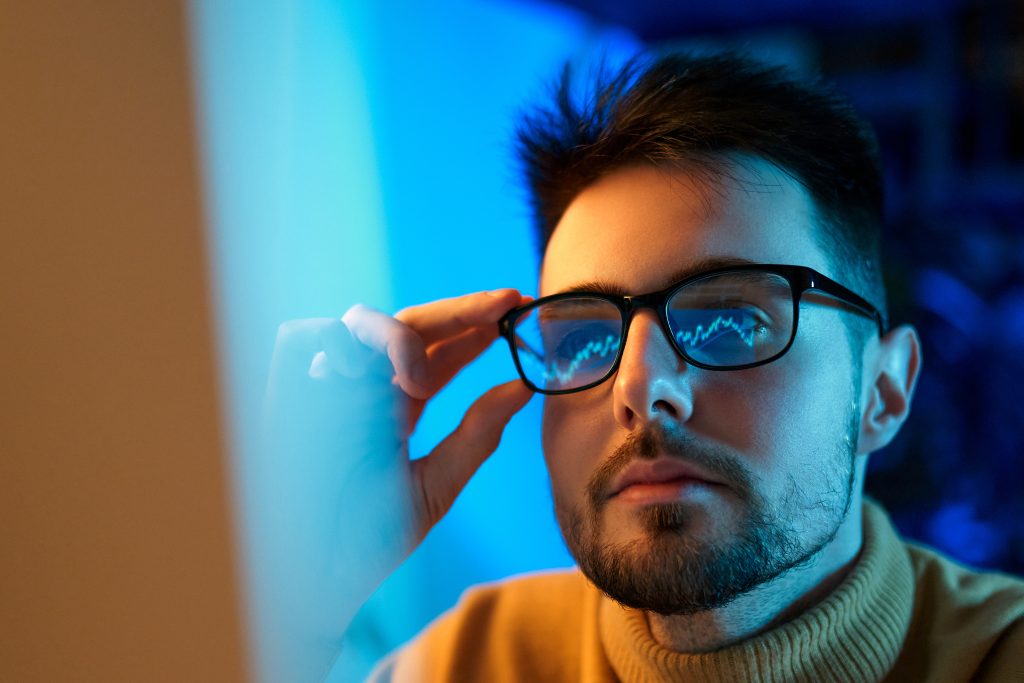
Most companies allocate a lot of money to ensure data quality in their projects. Having the right mix of good information and qualified data sources leads businesses to make informed decisions that will offer high returns on their investment.
A high-quality data set facilitates powerful decision-making in the following ways:
Agile Decisions
In today's fast-paced market, businesses must adapt their strategies according to the constantly evolving needs of consumers. With this in mind, gathering current and accurate data is essential. Using high-quality data gives businesses the confidence to make the right decisions in a consumer-centric marketplace.
Cohesive Results
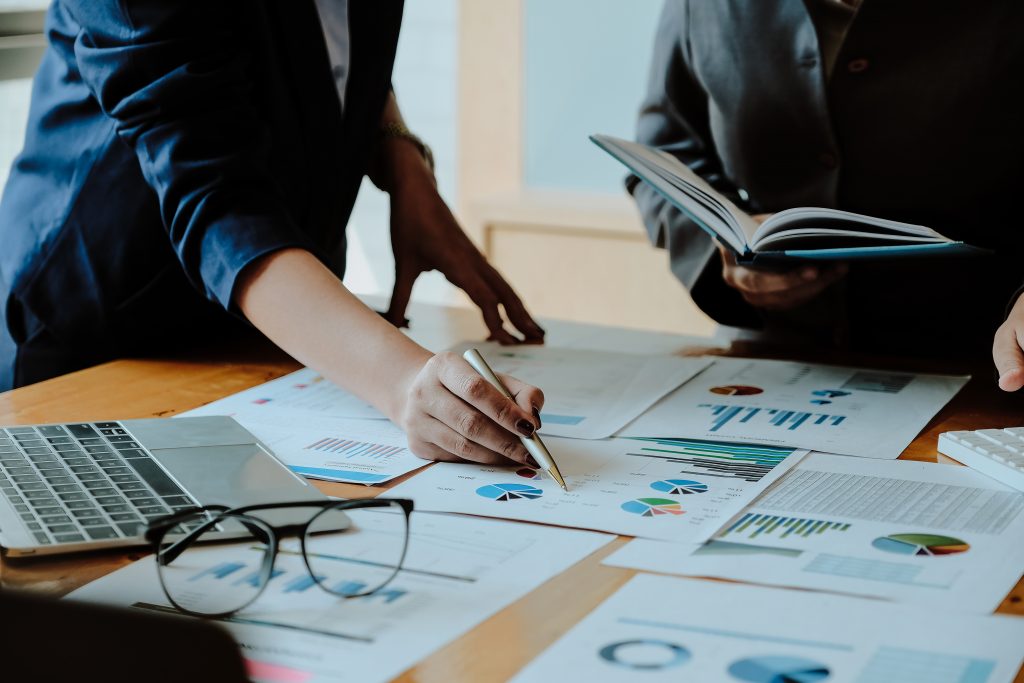
Inside companies that market to product buyers, multiple departments rely on consumer data to influence their decisions. By giving different teams access to high-quality data, companies can adopt a cross-functional approach that can help squash data silos while reducing communication errors between technical and non-technical teams. As a result, teams can stay aligned on priorities, branding, and messaging to yield more strategic and cohesive results.
Audience Targeting
Collecting high-quality data provides in-depth insight into your audiences’ needs, interests, and motivations. These findings give businesses direction, allowing them to focus their efforts on a high-potential target. Getting a holistic view of the customer helps organizations build better B2C relationships while developing products and campaigns that fit their market. with the objectives of your clients. With your expertise and experience in the market research industry, you can provide tailored recommendations that consider the broader context of the business and its goals.
IV. What is a Data Quality Check?
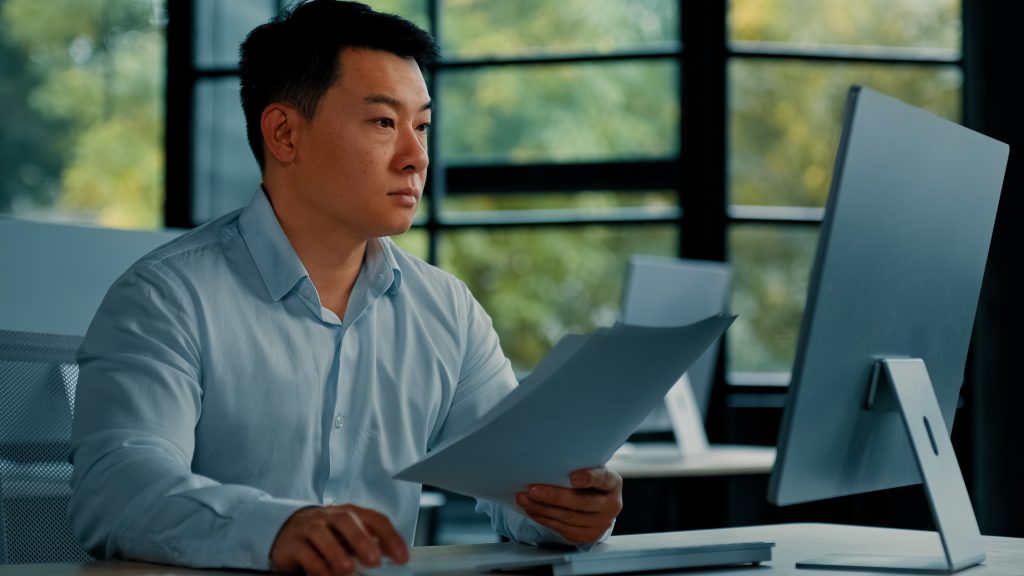
Data quality checks are an essential part of any research project. This involves thoroughly evaluating your methods to ensure that the data collected is trustworthy.
In qualitative research, rigor is a way to build trust in your findings. Since qualitative data tends to be varied and socially constructed, assessing rigor allows you to determine the reliability of your methods for capturing, organizing, and analyzing data.
This helps you learn how to establish structure and consistency in your methods over time. You can use these four types of data quality checks to appraise the rigor of your study: credibility, confirmability, dependability, and transferability.
Credibility
Credibility asks you to evaluate if your results represent truthful information drawn from respondents’ original feedback. Does your data accurately interpret what they originally meant with their responses? This criterion works well with experiences that are richly and accurately described.
The following methods are used to establish credibility:
- Prolonged Engagement: Investing long periods in observation or engaging with participants. This method allows you to familiarize yourself with the context of the study, test for misinformation, and build trust for richer data-gathering.
- Persistent Observation: Since qualitative data can vary depending on the context, frequently interacting with participants gives you an in-depth understanding of your data because any variances in answers would have already been accounted for.
- Triangulation: Using secondary methods or converging data from different sources to test the credibility of primary data.
- Debriefing and Peer Scrutiny: Consulting colleagues (e.g., an expert panel, project directors, a steering group) so they can examine, verify, and offer fresh perspectives on your findings.
- Negative Case Analysis: Discussing data points opposing the meaning you’ve interpreted from your data to look for alternative explanations that strengthen the ‘typical’ case.
- Referential Adequacy: Isolating a data segment while analyzing the rest and then comparing the themes identified with the isolated segment to look for similarities.
- Member Checking: Following up with participants, asking clarifying questions, and having them review your findings to ensure that their responses are accurately interpreted.
Confirmability
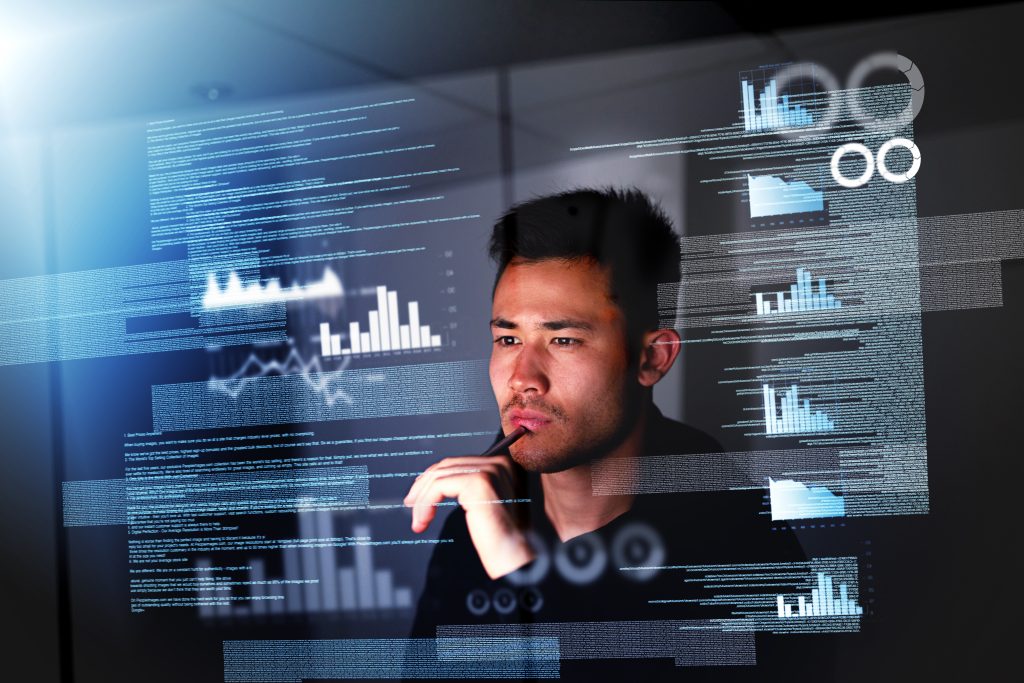
This criterion focuses on the researcher's objectivity or neutrality. Confirmability is established when your findings are grounded in the data and not based on personal preferences or viewpoints.
While achieving neutrality is quite challenging, some methods can help demonstrate how your identity influences your perceptions on certain topics and, in turn, how you interpret responses.
These include:
- Reflexivity: Acknowledging and confronting your biases in advance.
- Confirmability Audit: Sharing your research findings with colleagues to ensure that your interpretations aren’t unique to your perceptions.
Transferability
Transferability concerns the concept of applicability. As the name suggests, transferability is established if readers consider your findings applicable (or transferable) in their setting. This is done by describing the phenomena, its scope, and your process in rich detail.
Here are six questions you can use to describe the context of your data:
1. How many organizations participated in the study, and where are they based?
2. Are there any restrictions on the type of people who contributed their data?
3. How many respondents were involved in the fieldwork?
4. What were the data collection methods employed?
5. How many data-gathering sessions were conducted, and how long did they last?
6. Over what period was the data collected?
Dependability
Dependability is comparable to the concept of reliability in quantitative research. This criterion focuses on the transparency and consistency of your methods. It’s defined as the extent to which your study could be replicated in similar conditions.
To determine the dependability of your study, these types of data quality checks can be implemented:
Inquiry Audit: Involves having an external researcher evaluate the data collection and analysis process as well as research findings. All interpretations are examined to identify if your data supports them.
Audit Trail: Auditors examine records (e.g., field notes) of how the qualitative study was conducted. This helps them trace the researcher’s thought process throughout the study to determine whether the data is reliable.
V. Challenges that Affect Data Quality
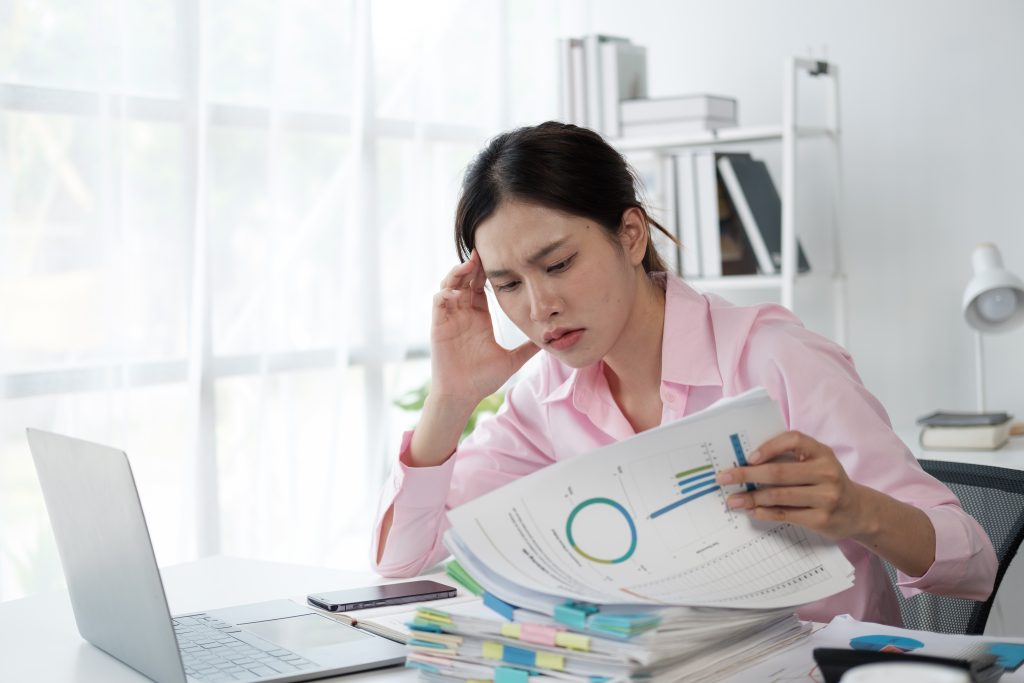
Although data quality is crucial in research, some problems may occur without a well-constructed research design. Quantitative methods such as surveys are prone to respondent fraud or click farm attacks that make your research invalid.
Meanwhile, qualitative methods that are open to interpretation run the risk of bias, incorrect interpretation, and disinformation. Data may also be gathered from unreliable sources or small samples that don't accurately reflect the population. These challenges can significantly impact data quality.
As such, you need to be aware of these data quality issues so you can properly address them. Here are some challenges you may run into when conducting a qualitative study.
Sampling-Related Challenges
Sample Size and Representation
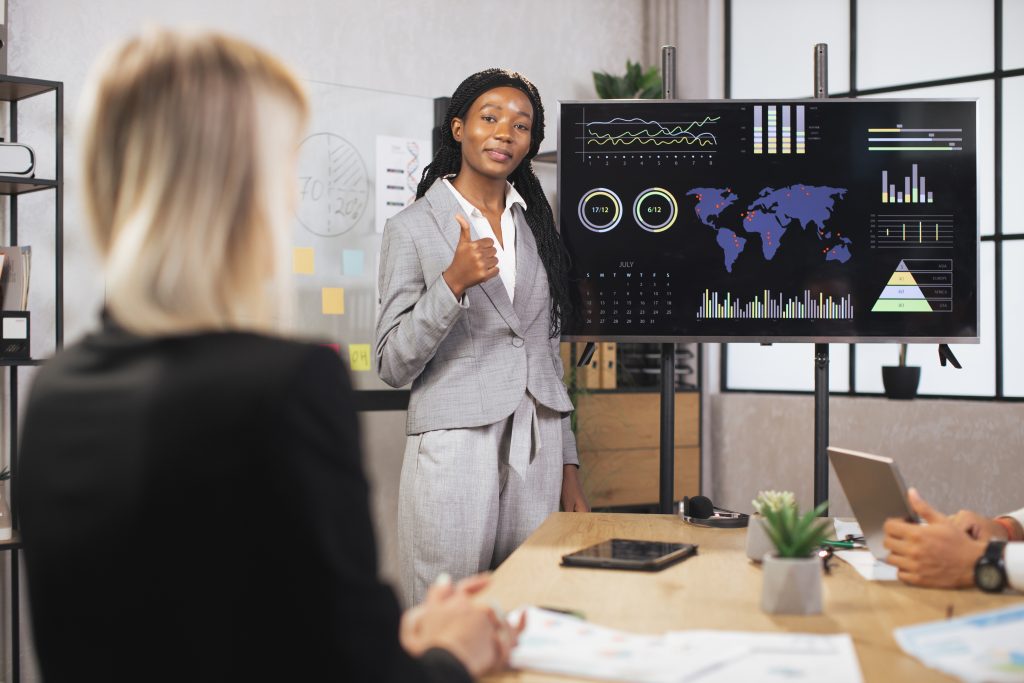
Qualitative sampling differs from quantitative sampling in various ways. Qualitative research uses non-probability methods wherein respondents are selected based on non-random criteria such as availability or geographic location.
Furthermore, qualitative research has a smaller sample size than quantitative research for detailed and intensive data-gathering. However, these two sampling issues present a few challenges that could affect your data quality. These include:
- Inaccurate representation of population: Does your sample accurately represent the population you want to study? It’s hard to determine if 10 or 20 people are typical of a large population.
- No set number of participants: How would you know if your sample size is too small or too large?
Unqualified Respondents
One of the biggest problems when collecting data is ensuring you're talking to the right people. In qualitative research, a qualified respondent is characterized by two things – articulation and authenticity.
Whether you’re doing in-person or online studies, we’ve all had groups wherein some respondents weren’t very helpful or didn’t seem to fit in with the group. But these issues are only noticeable during the session when it’s too late to exclude the participant.
This presents a few problems that could invalidate your data, such as:
- Skewed results: One bad respondent can influence other people's responses in the group. This false consensus leads the whole group to be invalid.
- Loss of client’s trust: If your clients see that you’ve recruited bad respondents, it could make them question the quality of the whole study. This is especially true if your findings don’t align with the client’s expectations.
Bias-Related Challenges
Respondent Bias
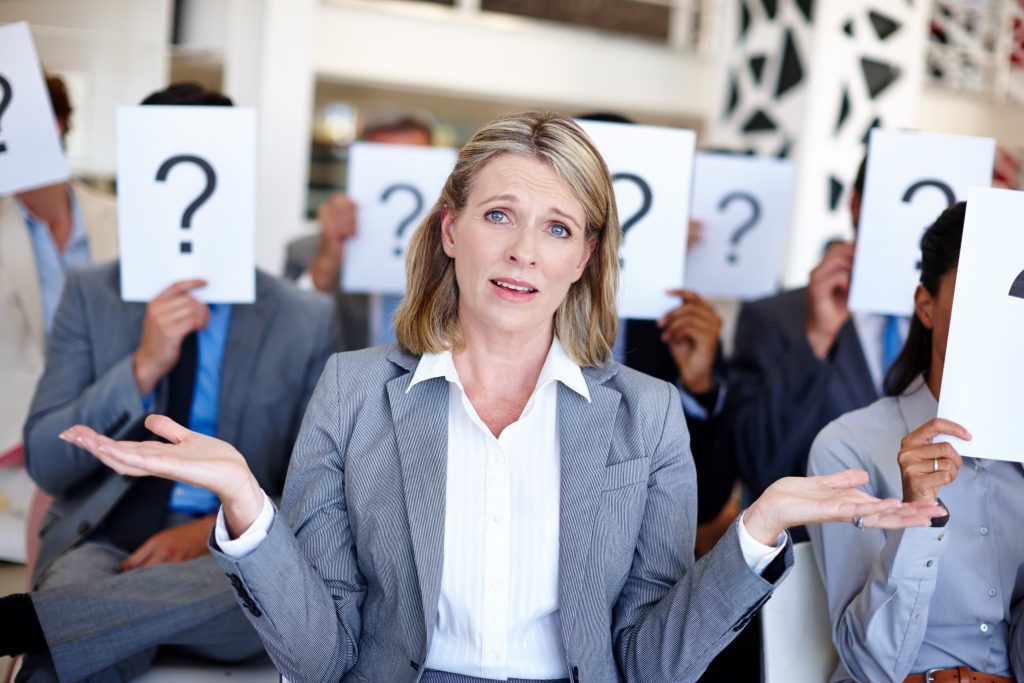
Respondent bias refers to any situation wherein your participant’s responses do not accurately reflect their true thoughts or feelings. This type of bias is composed of three types:
- Social desirability bias: Participants voice their answers regarding sensitive or controversial topics in a socially acceptable manner to avoid judgment.
- Agreement bias: Participants change their responses into what they think is the right answer to please the interviewer or moderator.
- Sponsor bias: Participants’ responses can be influenced based on their opinionated views about the research sponsor if revealed in the study.
Researcher Bias
This type of bias in qualitative research occurs when you intentionally or unintentionally manipulate your process or data in favor of a specific outcome. Various types of researcher bias include:
Question-wording bias: Asking questions that lead respondents toward one particular direction.
Confirmation bias: Interpreting data in a way that supports your hypothesis while also excluding any unfavorable data.
Question-order bias: Arranging research questions in a way that influences how the questions would be answered
Cultural bias: Formulating conclusions by looking at data through your own cultural lens
Halo/Horn effect: Making assumptions about a respondent based on a positive or negative attribute you’ve observed
VI. AI Ethics in Data Quality
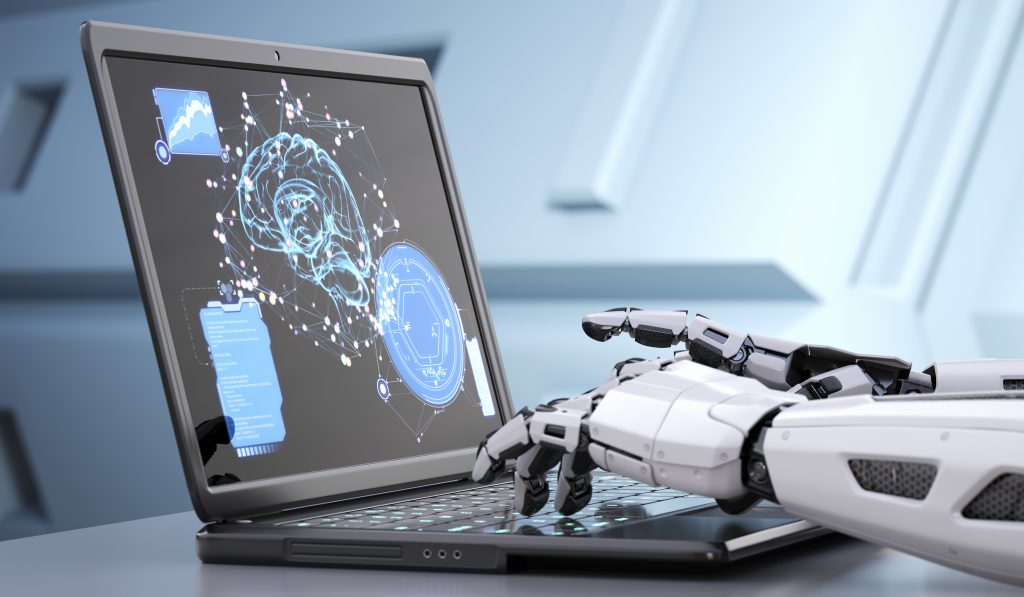
AI-driven market research has significantly reduced the amount of time it takes to execute each stage your workflow. However, it's important to consider the ethical considerations that come with this technology. In this section, we address AI ethics in qualitative research and provide recommended approaches for maintaining transparency, accuracy, and data security.
Most Common Ethical Concerns with AI Research
AI offers powerful capabilities, but as with any tool, its use must be governed by ethical considerations. Ensuring data quality with AI involves addressing these four ethical concerns:
- Data Privacy and Security
Data privacy remains a paramount concern in AI-driven market research. AI systems handle vast amounts of sensitive information, raising risks of data breaches and misuse. According to Pew Research, 53% of Americans believe AI is doing more harm than good regarding personal data privacy.
To safeguard respondent information, market researchers must adopt strict privacy protocols, including data compartmentalization and encryption, ensuring that sensitive data is secured and anonymized.
- Using AI as a Replacement for Human Analysis
One of the most common concerns with market research AI tools is if the technology will replace human expertise. AI demonstrates competence in identifying patterns and nuance, which are crucial in qualitative research, but it lacks empathy, cultural understanding, and the ability to grasp nuances.
Therefore, AI can serve as a tool to augment human analysis, but not replace it. A hybrid approach, where human subject matter experts review and validate AI-generated content, is essential to ensure depth and reliability.
- Training Data vs. Proprietary Content
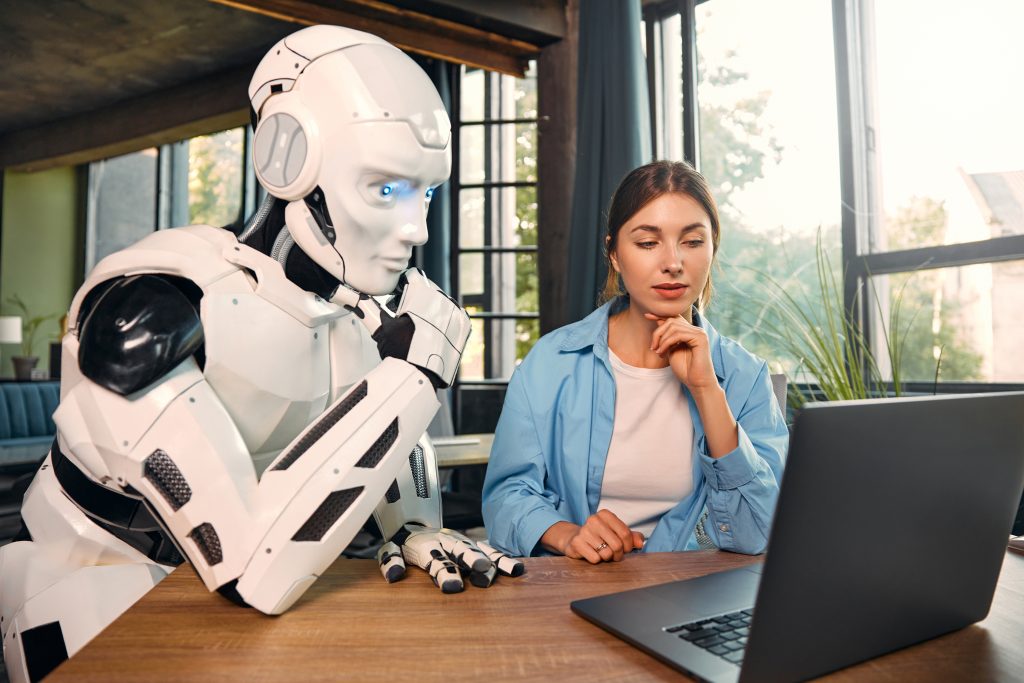
Transparency in how AI models use training data is essential. Companies must ensure that proprietary data isn't being used to train AI systems without explicit consent.
The rise of regulations like the EU AI Act or the recently introduced AI CONSENT Act provides a framework to ensure AI models handle sensitive data with care, focusing on transparency and human oversight.
- AI Hallucinations
AI-generated insights are only as good as the data they are trained on. Hallucinations—instances where AI presents false information as fact—are a significant ethical concern. According to research, AI chatbots invent information up to 27% of the time. Therefore it is essential that research data be handled within a ‘walled garden,’ where it does not draw insights from data unrelated to a specific research study.
Cross-referencing AI-generated insights with credible data sources and human validation are key steps to mitigate these risks.
VII. How to Maintain Data Quality: Best Practices
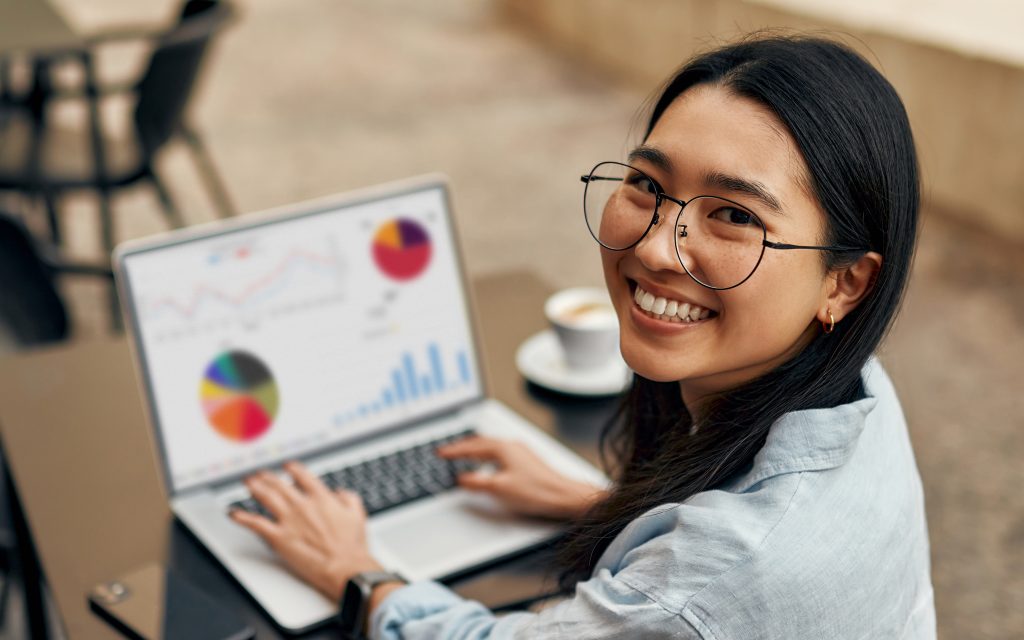
Maintaining data quality is important because no method is immune to sampling or bias-related challenges. Numerous types of data quality checks can be done to assess data quality and prevent bad data.
These practices account for different factors that direct exploratory research methods, such as focus groups or interviews. By using these methods in conjunction with each other, you can enhance the overall quality of your findings.
Best Practices for Sampling
Quota sampling: Divide your population into mutually exclusive subgroups (called strata) and recruit participants until your quota is reached. Each subgroup can be based on sex, age, social status, and criteria related to the topic under study, for example, job description or title, if the challenge is to understand product preferences for performing tasks related to job function. This gives you the best chance of recruiting a more representative respondent pool.
Aim for data saturation: You should keep selecting new respondents until data saturation is achieved, wherein the data brings nothing new. In practice, however, a methodological compromise is made, and most research proposals will set a certain number of groups and interviews. This allows budgets to be controlled and timetables to be developed.
Best Practices for Recruitment
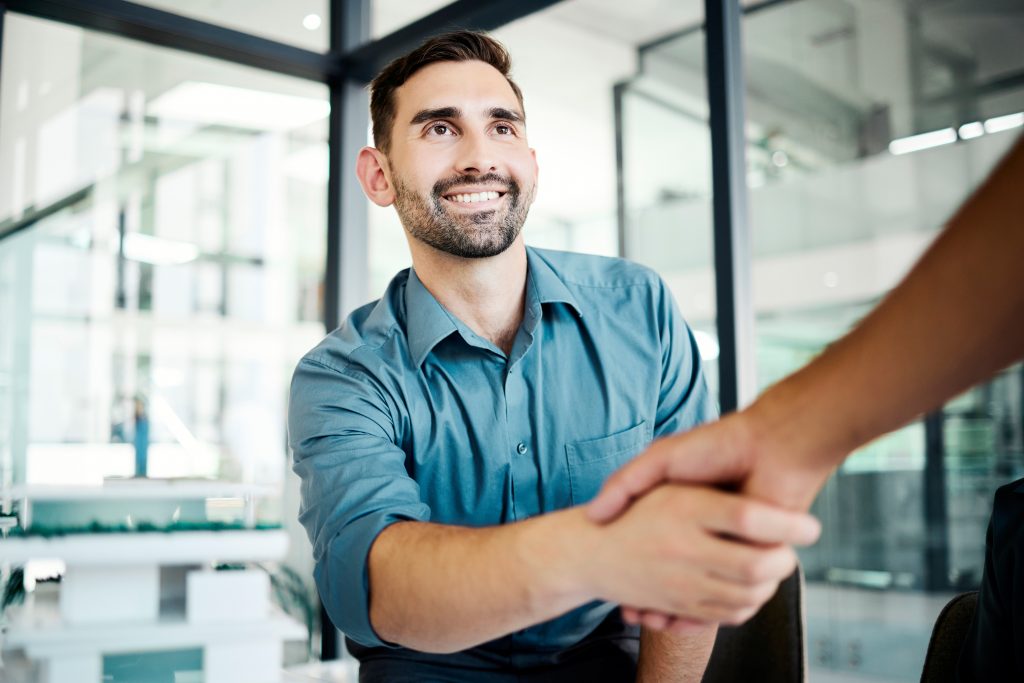
- Screening exercises: Have respondents perform a few tasks on camera, such as using a product or answering a few questions. Engaging in a video exercise before the project helps you gauge how clearlypotential respondents are able to communicate and if they are actually users of the product or service you're trying to investigate.
- Work with a reputed recruitment platform: Using a credible platform to find the right people for your study helps make the recruiting process less stressful and more efficient. Services such as CiviSelect™ offer respondent vetting capabilities plus screener review before respondent outreach.
Best Practices for Discussions
- Ask indirect questions: Frame your questions in a third-person perspective (e.g., ”What would he/she do?” instead of “What would you do?”). This prompts respondents to project their thoughts onto others – leading to more honest responses.
- Ask open-ended questions: Formulate and ask open-ended questions that encourage participants to reflect and expound on their responses for more detailed insight.
- Don’t give away client details: Client names or logos should be kept secret to prevent them from influencing respondent answers.
Best Practices for Data Analysis
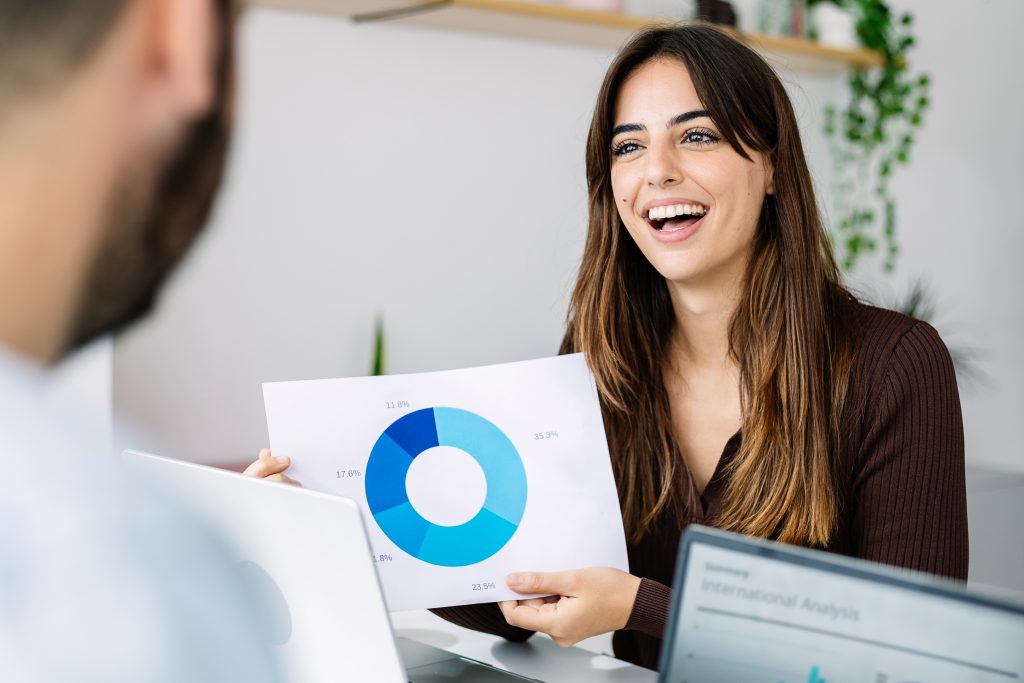
- Use multiple coders to interpret data: This strategy allows you to determine if your interpretation of the data is consistent with other people’s understanding.
- Conduct an external review of your work: Having a fresh pair of eyes to evaluate your findings helps you uncover bias-causing details (e.g., questions that need revision, gaps in argument) that you may have missed.
- Acknowledge your role in the study: Be critically self-reflective about how your personal identity could impact how you analyze data. Practice transparency when sharing your methods so readers can understand the logic behind your interpretations.
- Triangulate data sources: Have external sources (e.g., interviews, observations, document analysis) confirm your interpretations to know if the information you’ve gathered is legitimate.
- Use data analysis software: A qualitative data analysis tool helps extract actionable insights from the overwhelming amount of content generated in interviews and focus groups. It allows you to organize your data with ease so you can locate and analyze patterns in your findings.
Best Practices for AI Market Research
- Cross-reference insights: To ensure data quality, AI-generated insights must be cross-verified with trusted data sources.
- Continuous validation: Build a feedback loop wherein you flag instances where AI outputs were inaccurate or unhelpful. This process can inform future training cycles and improve the model’s performance over time.
- Human oversight: Incorporate human expertise at key points in the analysis process to mitigate biases and errors the AI research assistant tool might miss.
- Transparency in AI usage: Disclose AI’s role in the research process to stakeholders, ensuring ethical compliance.
VIII. Conclusion
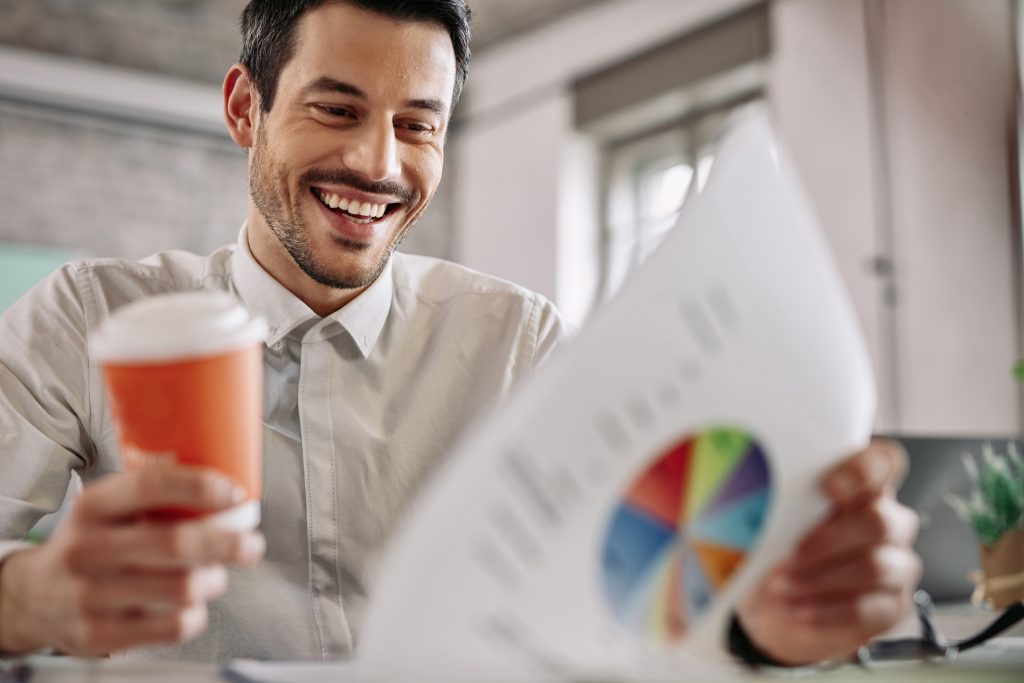
By adhering to a few principles, you can ensure data quality in your qualitative project. These include having a clear understanding of your research objectives, collecting and analyzing data in a systematic manner, maintaining consistency throughout the data collection process, and rigorously interpreting the data. Following these guidelines will help you obtain respondent data that delivers trustworthy information.
Gather High-Quality Qualitative Research Data With Civicom® Marketing Research Services
For over 20 years, Civicom® Marketing Research Services has been at the forefront of delivering exceptional online and in-person qualitative solutions to meet the diverse needs of market researchers worldwide. From respondent recruitment to project facilitation and unwavering technical support, we have you covered at every step. With an unwavering commitment to excellence, we have established ourselves as a global leader in providing end-to-end support that enables researchers to deliver qualitative project success.